Computer Vision Projects
Custom CPU for ML Experiments
Overview: Built a custom CPU from scratch specifically for running machine learning experiments.
Components Used:
- Motherboard: ASRock X670E TAICHI CARRARA
- CPU: AMD Ryzen 9 7000 series 16 core 32 thread
- GPU: Nvidia GEFORCE RTX 4090 24GB
- RAM: VENGEANCE LPX DDR5 64GB (2x32GB)
- Hard Drive: WD - BLACK SN850X 2TB
- Power Supply: Gamemax 1300w
- Cooling System: Hyper 212 Halo
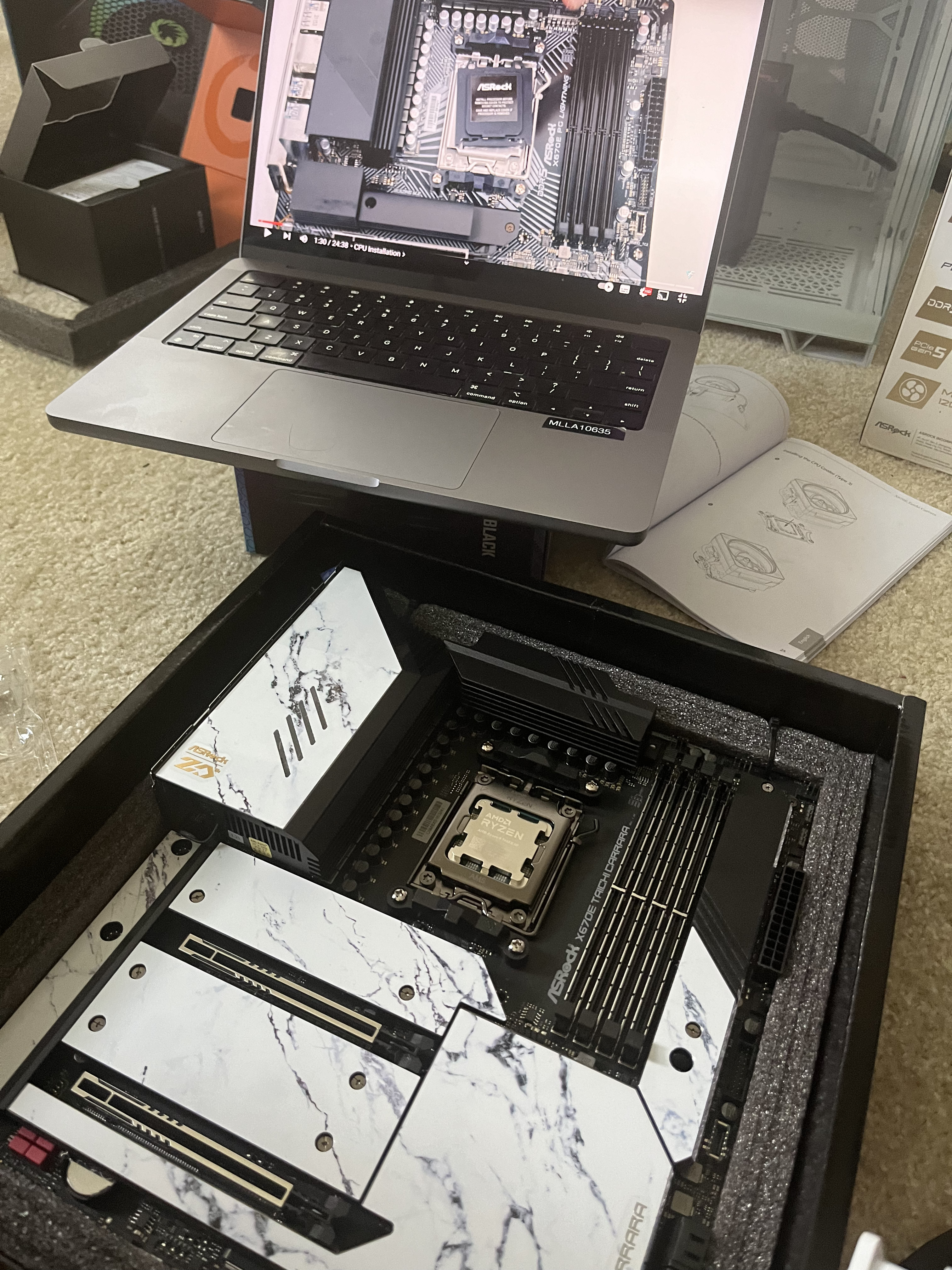
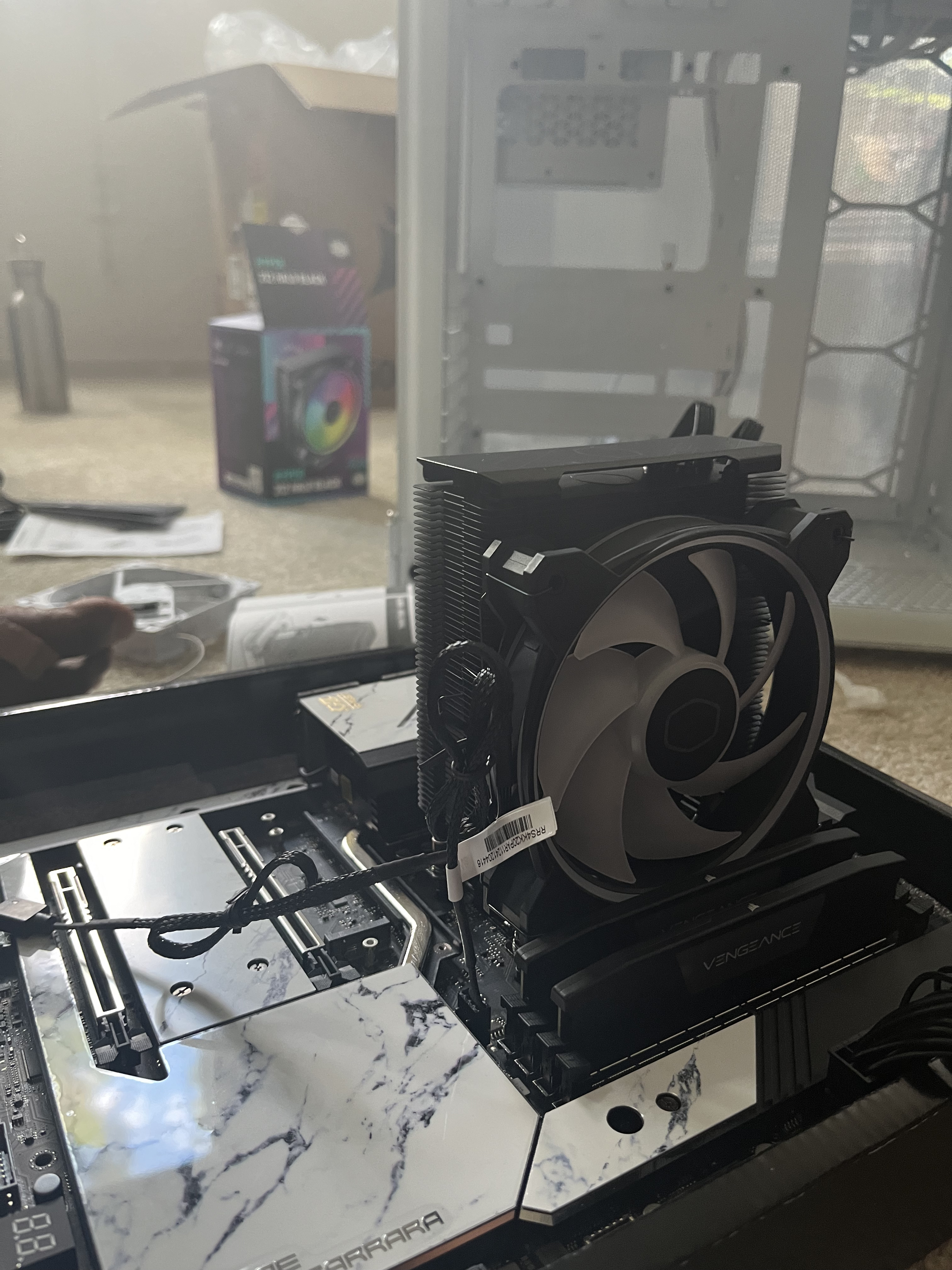
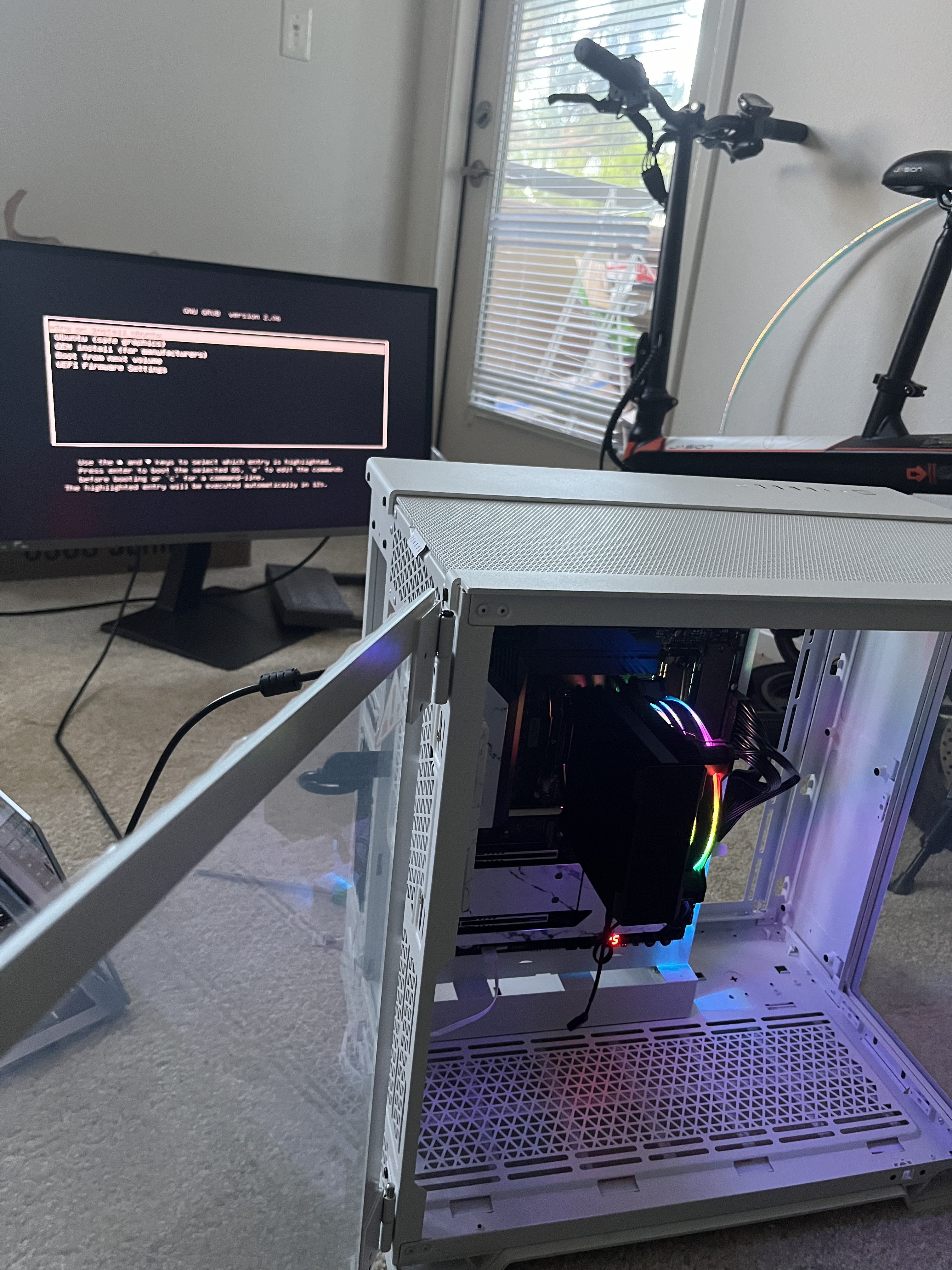
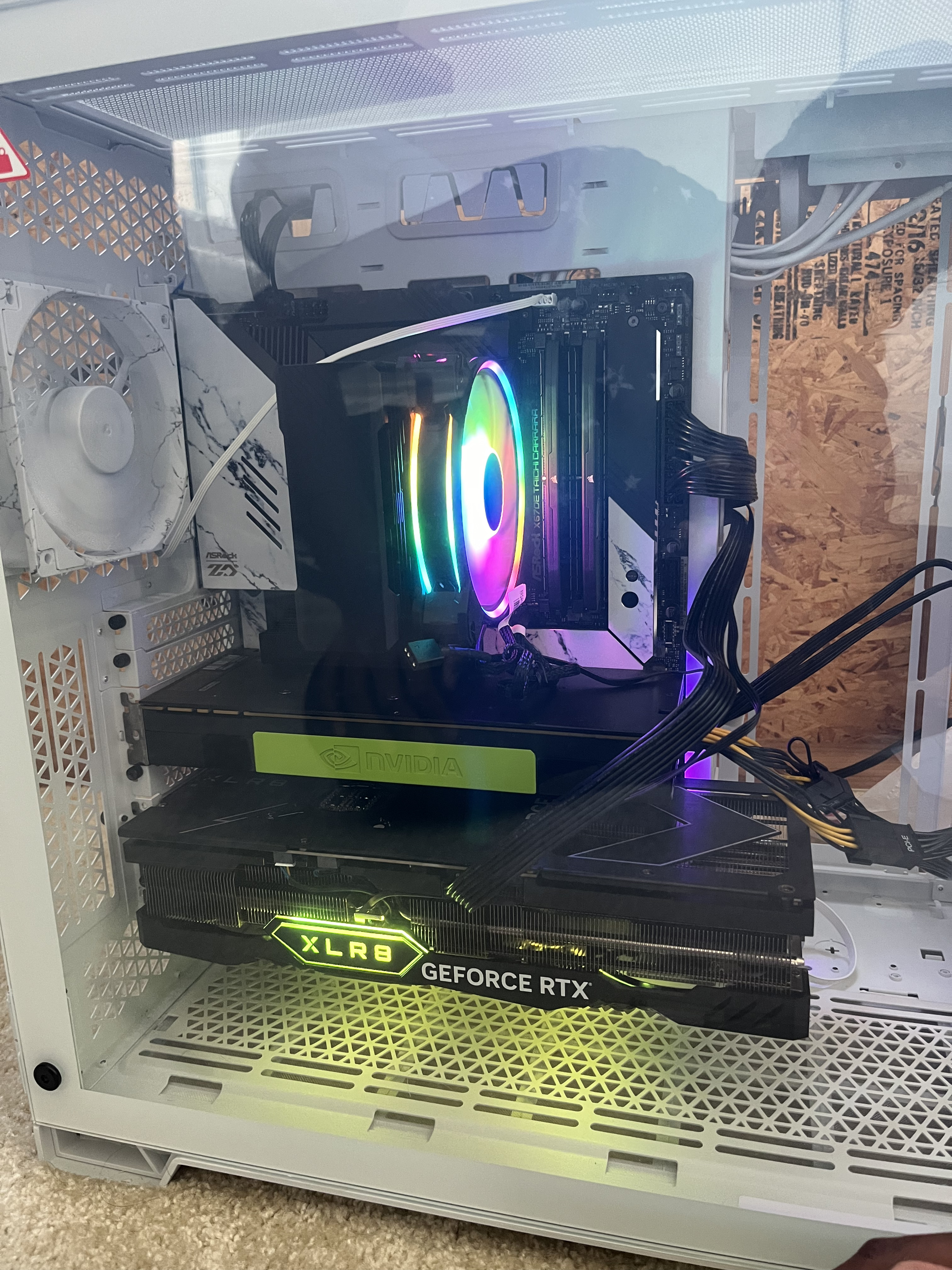
3D Model Reconstruction and Visualization Platform
- Transform Images to 3D Models: Upload 2D images to generate high-fidelity 3D reconstructions with ease.
- Point Cloud and Video Outputs: Backend processes images to create both PLY files and MP4 videos for visualization.
- Interactive 3D Viewer: Render
.glb
models dynamically in a WebGL-powered single canvas. - User-Friendly Features: Drag-and-drop uploads, real-time status monitoring, and options to download or share generated models.
- Modern Tech Stack: Built with Astro, React, FastAPI, Three.js, and Trimesh for optimal performance and scalability.
- Scalable Hosting: Deployed using AWS Amplify and Vercel to ensure reliability and speed.
- Simplified Workflows: Designed for an intuitive and hassle-free user experience in 3D reconstruction.
Discover the power of converting images into 3D visualizations like never before! Try the PointCloud App Here
Evaluating Perceptual and Geometric Fidelity of Text-to-3D Models
- Innovative Research: Co-authored a publication for CVPR 2025, titled “Evaluating Perceptual Fidelity of Text-to-3D Models”.
- Dual-Fidelity Assessment: Developed evaluation frameworks focusing on both perceptual fidelity (alignment with human perception) and geometric fidelity (accuracy of 3D structures).
- Multi-Modal Analysis: Combined insights from natural language processing, 3D geometry, and computer vision to establish comprehensive evaluation metrics.
- Advanced Testing Frameworks: Designed tools and workflows to measure structural and visual consistency in machine-generated 3D models.
- Collaborative Effort: Partnered with experts to push the boundaries of text-to-3D synthesis research.
- Impactful Results: Contributed to benchmarks that enhance model quality and bridge the gap between AI-generated 3D models and real-world expectations.
3D-Ready
Overview: The 3D-Ready application leverages a modern stack including React for the frontend and FastAPI for the backend, seamlessly integrated with various AWS services like Amplify, Lambda, S3, DynamoDB, and API Gateway.
Deployment: Utilizing AWS’s robust infrastructure, the application ensures high availability and scalability, offering users an efficient and reliable experience for generating and viewing 3D models.
Media Files and Links:
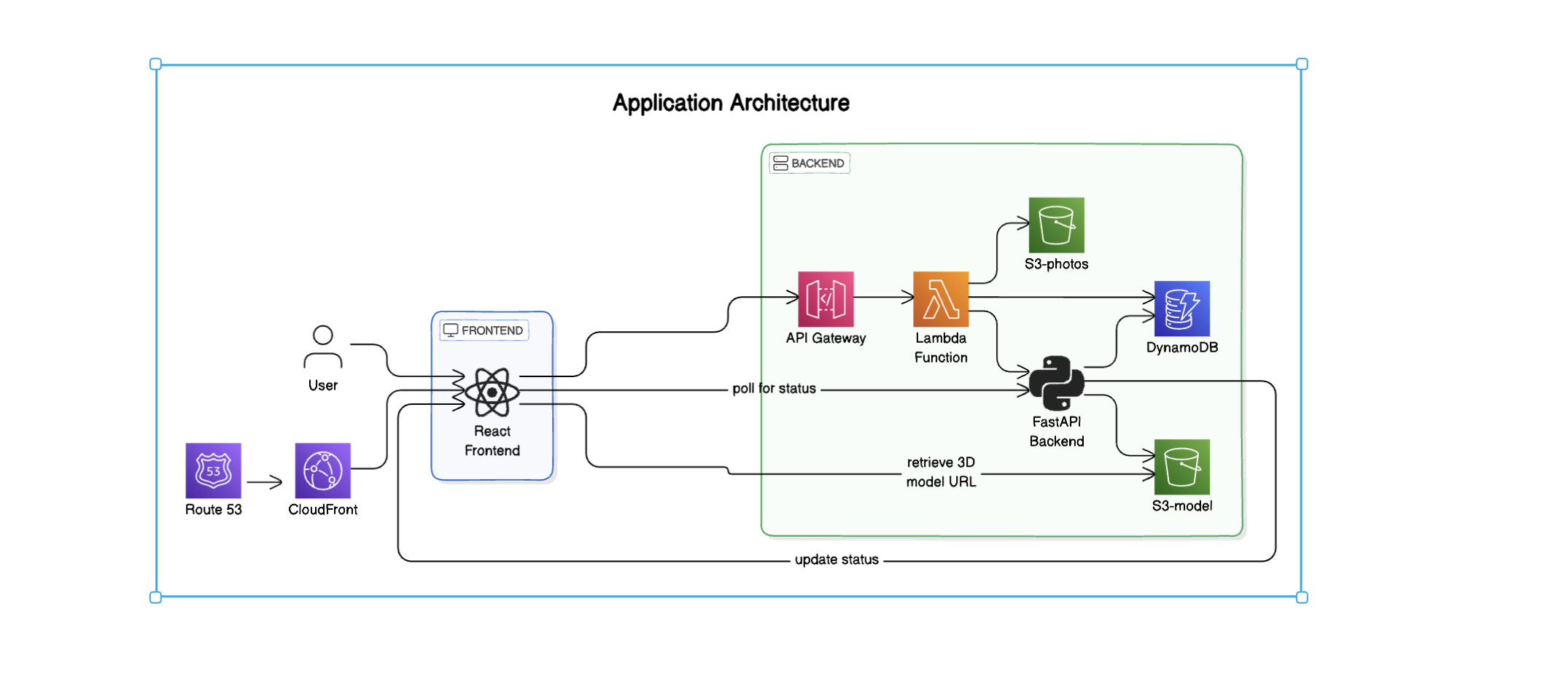
Experiment : Gaussian Splatting using Acezero
Overview: The goal was to experiment with camera calibration techniques for Gaussian splatting. I developed a data loader script to convert Acezero’s camera pose estimation and point cloud output into a format suitable for Gaussian splatting. I precisely handled homogeneous matrices to ensure accurate data transformation and alignment.
Media Files and Links:
Experiment : Efficient NeRO
Overview: The goal is to leverage Hash encoding (like InstantNGP) for geometry reconstrcution to achieve faster 3D representations of reflective objects.
Technical Approach: -
- Python: For scripting, data processing, and automation of the pipeline.
- PyTorch: As the deep learning framework to implement and train the NeRO model.
- CUDA: For GPU acceleration to enhance processing efficiency and speed.
Media Files and Links:
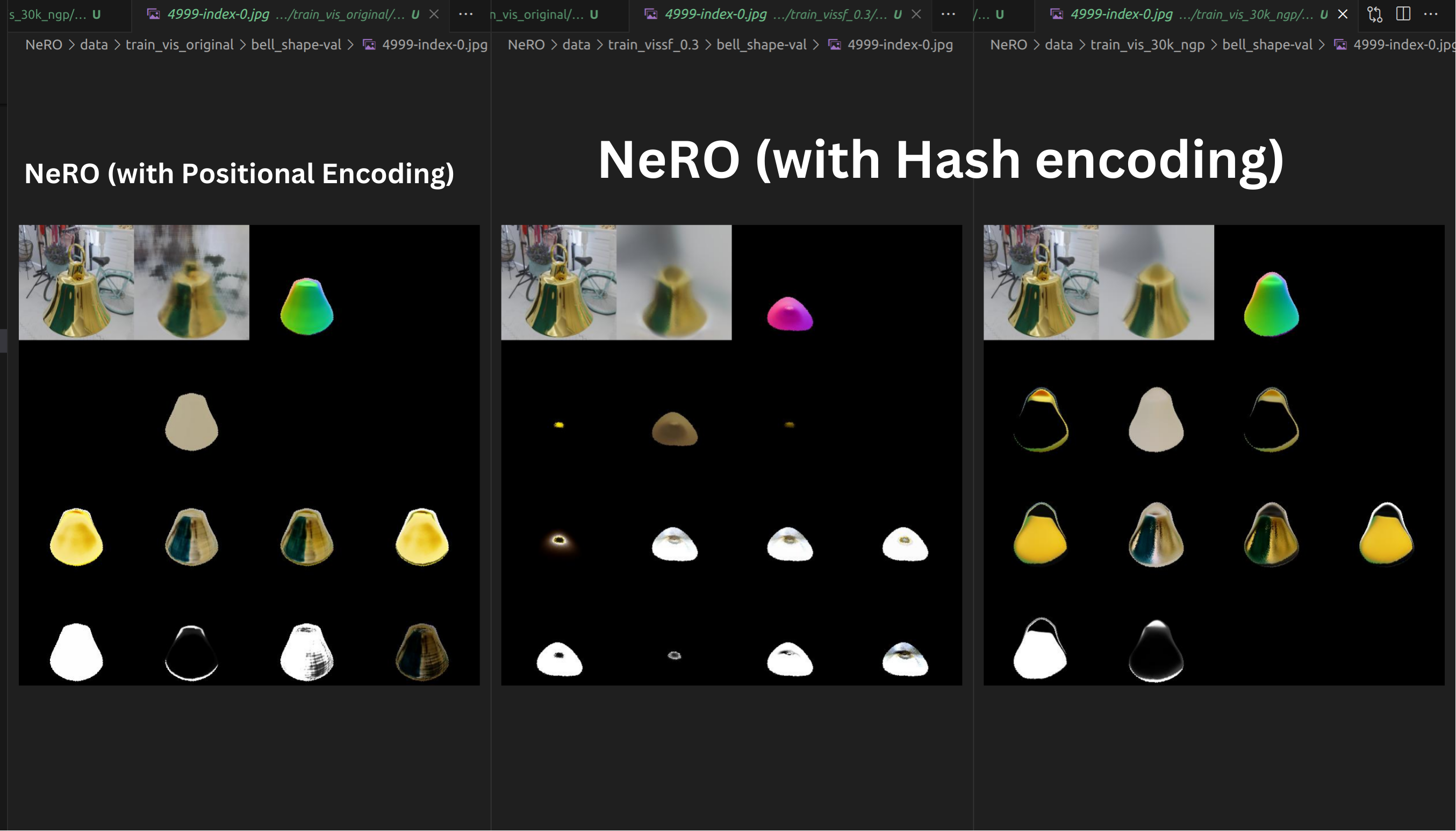
Experiment : Gaussian Surfels
Overview: This project involves experimenting with Gaussian Surfels
Media Files and Links:
Experiment : MVSplat
Overview: This project involves experimenting with MVSplat
Media Files and Links:
Experiment : NeRO Bell
Overview: This project involves experimenting with NeRO (Neural Geometry and BRDF Reconstruction of Reflective Objects from Multiview Images) to create a high-quality 3D model of a bell. The goal is to leverage NeRO’s advanced neural geometry and BRDF (Bidirectional Reflectance Distribution Function) reconstruction techniques to achieve precise and realistic 3D representations of reflective objects.
Technical Approach: -
- NeRO: For neural geometry and BRDF reconstruction from multiview images.
- Python: For scripting, data processing, and automation of the pipeline.
- PyTorch: As the deep learning framework to implement and train the NeRO model.
- CUDA: For GPU acceleration to enhance processing efficiency and speed.
- Blender : For Rendering
Experiment : NeRF Fox
Overview: This project creating a Neural Radiance Field (NeRF) of a fox using instant NGP. The result is a high-fidelity 3D representation of the fox that can be rendered from various viewpoints.
Technical Approach: -
- instant NGP: For generating the NeRF.
- Python: For scripting and automation.
- CUDA: For GPU acceleration.
Media Files and Links:
Experiment : Gaussian Splatting
Overview: This project involves creating a high-fidelity 3D representation of a landscape scene using Gaussian splatting. The result is a detailed and visually appealing 3D model that can be viewed from multiple perspectives.
Technical Approach:
- Gaussian Splatting: For generating the 3D model.
- Python: For scripting and automation.
- CUDA: For GPU acceleration.
Media Files and Links:
2D Image Fitting Using KAN (Kolmogorov–Arnold Networks)
Overview: This project involves fitting 2D images (MNIST) using Kolmogorov–Arnold Networks (KAN) to improve image representation and reconstruction.
Technical Approach: The project utilizes Kolmogorov–Arnold Networks for effective 2D image fitting, leveraging advanced mathematical techniques to achieve high accuracy in image reconstruction.
Media Files and Links:
Structure from Motion
Overview: This project focuses on the structure from motion (SfM) technique using Python, Numpy, OpenCV, and GPU to reconstruct 3D structures from 2D images.
Technical Approach: The SfM technique is implemented using Python and libraries like Numpy and OpenCV. GPU acceleration is utilized to enhance computational efficiency.
Media Files and Links:
- COLAMP SMF
- COLMAP POINTCLOUD
Image Classifier Using PyTorch
Overview: Rebuilt the OG PyTorch-based image classification project to accurately categorize images into predefined classes, demonstrating proficiency in deep learning and computer vision.
Technical Approach: Implemented a Convolutional Neural Network (CNN) with multiple convolutional and fully connected layers, utilizing ReLU activation, max pooling, and backpropagation for training, while leveraging the Stochastic Gradient Descent (SGD) optimizer and cross-entropy loss for efficient learning. Model trained on CIFAR10 datatset.
Media Files and Links: